Don't Learn How to Code*
*If you don't want to, you don't have to.
Our guide to code-free careers explores the professional opportunities where your mathematical skills can shine, no programming required. Discover how to leverage your expertise into a fulfilling career that could redefine your professional trajectory.

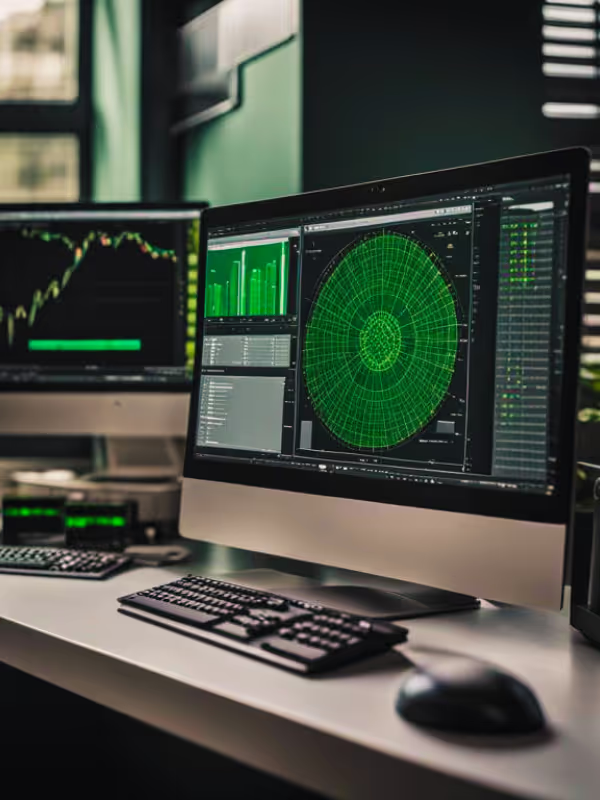

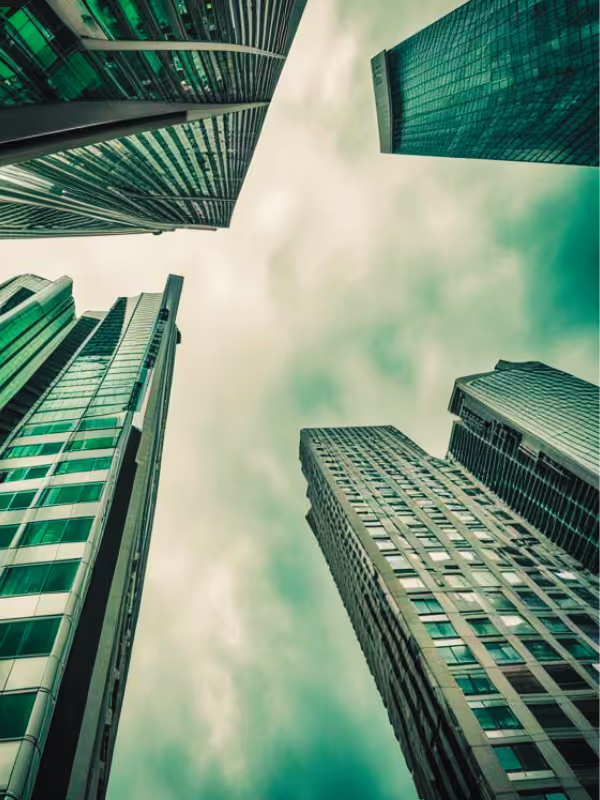

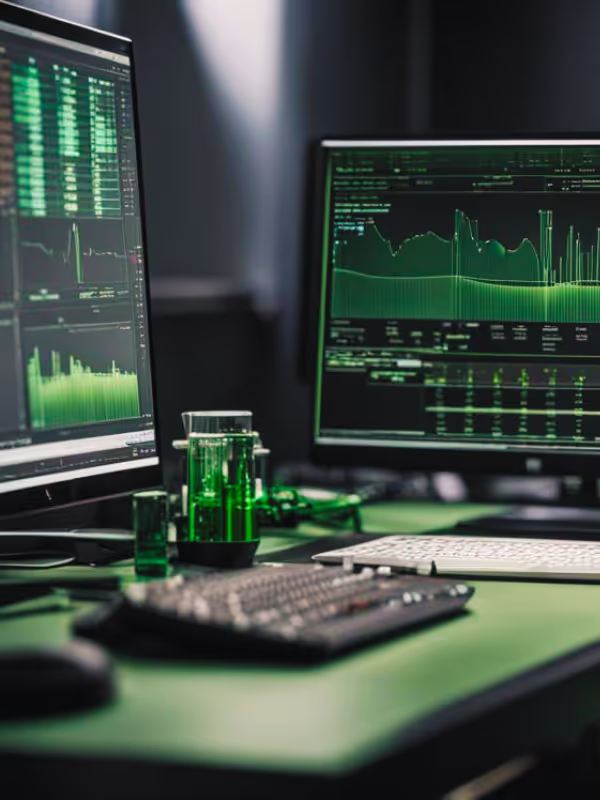

Code-Free Careers
Leverage your unique skills in a rapidly evolving job market, positioning yourself at the forefront of problem-solving and leadership — all without writing a single line of code.
Problem-Solving without Programming
The world of professional mathematics is diverse, offering a multitude of paths for those with mathematical expertise. While many mathematicians excel in coding and find fulfilling careers in data science, software development, and other technical fields, there's a growing recognition that coding isn't the only way to leverage mathematical abilities in the professional world.
The landscape of mathematical careers is evolving, driven by two key factors:
The mathematical skill set is extensive, involving strategic and operational competence as much as technical ability. (We've built a map of the mathematical skill set - you can find it here [link].)
The proliferation of platforms that automate many previously hard-coded tasks is making programming less essential for many roles.
By exploring non-coding career paths, we aim to see more mathematicians in positions of strategy and leadership. We also intend to collapse the timeline from degree to career, ensuring that no one feels compelled to accept a position beneath their skill level.
Moreover, adopting a problem-driven approach, rather than focusing solely on specific tools or coding languages, offers significant advantages in future-proofing your career. This flexibility allows you to adapt to new technologies and methodologies as they emerge, maintaining your relevance in an ever-changing job market.
To support your journey, we've compiled a collection of over 20 nontechnical and no-code career tracks well-suited for those with strong mathematical backgrounds. In this guide, we'll focus specifically on no-code career tracks: roles where analytical platforms and other tools are used to implement and apply mathematical knowledge without the need for traditional programming.
Guide Contents
This guide is dedicated to those mathematicians who either don't want to code or prefer not to make it a central part of their career. Whether you're skilled in coding but seeking a different direction, or you've never written a line of code and don't intend to start, there are numerous opportunities to apply your mathematical knowledge and skills without programming.
Whether you're a recent graduate, changing careers, or simply exploring your options, this guide will show you how to leverage your mathematical might in the professional world, all without writing a single line of code.
Why Consider Nontechnical & No-Code Roles?
By choosing the no-code route, you're not just finding an alternative to coding – you're opening up new possibilities for applying your mathematical skills in innovative and impactful ways. In today's data-driven world, almost any role can be a "math role."
No-code platforms are making it easier for businesses across all sectors to harness the power of data and analytics. As a result, your mathematical expertise will be valuable in unexpected places, opening up a wider range of career possibilities. Other benefits include:
Skip the time-consuming and expensive coding bootcamps. With no-code options, you’ll be able to start applying your mathematical skills to real-world problems right away, without spending months and thousands of dollars learning to code.
Rather than getting bogged down in specific coding languages or tools that may become obsolete, you can concentrate on what truly matters: solving complex problems. This approach can allow you to apply your mathematical thinking more directly to challenges in various industries.
By pursuing no-code and nontechnical options, you can focus on applying the deeper mathematical skills that truly fulfill you intellectually and professionally. This alignment between your work and your strengths can lead to greater job satisfaction and career growth.
Overview of No-Code and Low-Code Platforms
In today's data-driven business environment, three main categories of analytical platforms have emerged as essential tools across diverse industries.
From cutting-edge startups to established corporations, and spanning sectors as varied as space technology, biotechnology, software development, and everything in between, these platforms offer core functionalities required by most modern enterprises. They correspond to roles that are analytical in nature but not overly technical, making them ideal for mathematicians seeking to apply their skills without deep coding expertise.
Most of these platforms are designed with no-code interfaces, allowing users to harness powerful analytical capabilities through intuitive, visual interfaces. Some platforms may fall into the low-code category, requiring minimal programming knowledge. However, this shouldn't deter mathematicians, as the learning curve is generally manageable and the payoff in terms of analytical power is substantial.
AI & ML Platforms
ML & AI platforms democratize access to cutting-edge technologies, allowing users to harness the power of AI without deep technical expertise. These integrated environments enable users to build, train, and deploy machine learning and artificial intelligence models, offering tools for data preprocessing, model development, and predictive analytics.
Caveat: While these platforms offer powerful capabilities, it's important to note that traditional coding and data science approaches still permit more rigor and customization. However, no-code AI & ML platforms excel in more straightforward use cases and routine analytics, making advanced technologies accessible to a broader range of professionals.
- Prebuilt Models and Templates: with extensive libraries of prebuilt models and templates (ranging from text analysis to complex deep learning models), these platforms can empower users to quickly deploy sophisticated AI functionalities while significantly reducing development time.
- Data Preparation and Processing: these platforms provide intuitive interfaces and functionalities to manage large datasets, perform exploratory data analysis, and prepare data in formats suitable for diverse machine learning algorithms, including capabilities for data cleaning, data integration, and feature engineering.
- Model Training and Evaluation: these platforms allow users to adjust parameters, iteratively improve models based on feedback, and fine-tune models to suit specific business needs. They also help users evaluate performance using metrics such as accuracy, precision, and recall, among many others.
- Model Deployment and Hosting: with capabilities for both model deployment and hosting, these platforms can simplify the often complex process of bringing AI models live. They provide tools for deploying into various production environments, including cloud-based and on-premises infrastructures.
- Model Monitoring and Management: to provide a comprehensive view of AI model performance post-deployment, these platforms often offer continuous oversight, tracking model performance metrics, detecting issues such as drift or degradation over time, enabling retraining, and managing model versions to ensure models remain accurate in dynamic real-world conditions.
- Explainability and Transparency: in environments in which compliance with regulations is mandated or transparency is needed, these features allow businesses to gain insights into the inner workings of complex algorithms.
- Scalability: this fundamental feature ensures these systems can handle increasing data volumes and growing analytical demands as businesses expand and data complexity grows, maintaining high-performance levels.
- Smart Grid Optimization using Predictive Analytics: an energy company uses time series forecasting to predict energy demand and optimize the distribution of electricity in smart grids, enhancing energy efficiency.
- Space Exploration Data Analysis with AI: a space agency employs convolutional neural networks to analyze data from telescopes and space — vast amounts of imagery and sensor data from space missions — to identify celestial phenomena.
- Material Discovery with Bayesian Optimization Models: a material science company applies Bayesian optimization models for predicting the properties and behaviors of new materials, accelerating the discovery process.
- 3D Modeling in Engineering using Generative Adversarial Networks (GANs): an engineering firm uses GANs for creating sophisticated 3D models and simulations, improving design accuracy.
- Robotic Automation in Manufacturing using Reinforcement Learning: a manufacturing company integrates reinforcement learning with robotics for dynamic and adaptive automation on production lines.
- Advanced Video Analysis using Object Detection Models: a security firm employs object detection models for analyzing surveillance footage, accurately identifying and tracking objects and activities.
- Agricultural Yield Prediction using Random Forests: Agritech firms use random forest models to analyze climatic and soil data, predicting crop yields with greater accuracy and aiding in agricultural optimization.
- Quantum Computing Algorithm Optimization with Reinforcement Learning: researchers in a quantum computing startup use reinforcement learning to optimize quantum algorithms, improving computation efficiency.
- Genetic Data Analysis for Personalized Medicine using AI: a biotech firm applies genetic algorithms to analyze genetic data, enabling approaches tailored to individual genetic profiles.
- Cybersecurity Threat Detection with AI: a cybersecurity firm uses anomaly detection models to analyze network traffic and detect anomalous patterns, predicting and preventing cyber attacks in real time.
Data Scientists, Machine Learning Engineers, AI Researchers, Data Engineers, Robotics Engineers, Bioinformaticians, Computer Vision Engineers, NLP Specialists, and more.
Builder.ai, Squark, UiPath, DataRobot, Botmock, BigML, GeneXus, Obviously.ai, Arize, LatticeFlow, WhyLabs, Lightning, Mosaic, MindsDB, Replicate, Credo AI, Holistic AI, Cohere, Hugging Face
Analytics Platforms
Analytics platforms provide user-friendly interfaces for data exploration, visualization, and interpretation. These tools empower mathematicians to leverage their analytical skills without writing complex queries or scripts.
From interactive dashboards to automated reporting, these platforms allow users to track key performance indicators (KPIs), predict future market trends, understand customer behavior, and more. These platforms streamline data operations, saving time and resources and allowing users to uncover valuable business trends and opportunities.
- Advanced, Self-Service Analytics: these platforms offer a range of powerful tools including regression models, classification algorithms, and forecasting techniques which can be used to analyze historical data as well as predict future scenarios, all through intuitive, user-friendly interfaces that require minimal to no coding.
- Business Intelligence: users can easily create comprehensive reports, conduct market analysis, and monitor KPIs (key performance indicators), utilizing features such as drag-and-drop (no-code) report builders and customizable dashboards, all designed for ease of interpretation.
- Data Visualization and Reporting: these platforms offer sophisticated data visualization capabilities, allowing for the creation of detailed, customizable, and interactive reports and dashboards that bring data to life. Users can easily design dashboards that highlight key metrics and trends, employing a range of visualization styles – from bar charts to heat maps.
- Data Integration and ETL (extract, transform and load): these platforms offer robust ETL tools that automate the process of aggregating data from multiple sources, including cloud services, databases, and external applications. Users are able to integrate disparate data easily, clean it and organize it efficiently, and prepare it for analysis.
- Data Collaboration and Sharing: users are provided with tools for secure data sharing, version control, and collaborative editing. This helps to ensure data consistency and accuracy while pooling expertise from different departments.
- Data Governance and Compliance: users have access to mechanisms such as data security protocols, access permissions, and audit trails that help organizations maintain data integrity and adhere to legal compliances.
- Customization and Scalability: these platforms offer features that help users adapt them to the evolving needs of the business, accommodating growing data volumes and changing analysis requirements. They provide scalable architectures and the ability to be customized to fit unique business contexts.
- Supply Chain Disruption Analysis with Risk Assessment Models: a manufacturing company uses risk assessment models in their business intelligence platform to evaluate and predict supply chain vulnerabilities, allowing proactive measures to be taken to mitigate disruptions.
- Energy Consumption Analytics with Regression Models: a corporation implements regression analysis to correlate energy consumption with operational factors across facilities, identifying key drivers of energy use for targeted sustainability efforts.
- Predictive Maintenance using IoT Sensor Data Analytics: a manufacturer integrates IoT sensor data with predictive analytics models to anticipate equipment failures, scheduling maintenance before breakdowns occur.
- Public Transportation Route Optimization with Simulation Models: a city’s transportation department uses simulation models to test and optimize bus and train schedules, improving punctuality and passenger distribution.
- Pharmaceutical R&D Prioritization with Decision Trees: a pharmaceutical company implements decision tree analysis to prioritize R&D projects, evaluating market potential and development feasibility systematically.
- Educational Program Analytics with Cohort Analysis: a university applies cohort analysis to track and compare student performance over time, tailoring educational programs to improve learning outcomes.
- Resource Allocation Analysis with Linear Programming: a quantum computing firm utilizes linear programming models to optimize resource allocation for R&D projects, balancing different initiatives based on resource availability and project priority.
- User Experience Feedback Analysis with Sentiment Analysis: an XR company employs sentiment analysis algorithms to parse through user feedback, identifying key themes and sentiments about usability and experience across various extended reality applications.
- Customer Usage Pattern Analysis with Cluster Analysis: a satellite data services company uses cluster analysis, segmenting clients into groups based on usage behaviors to tailor services more effectively to different market needs.
Data Analysts, Business Intelligence Analysts, Product Analysts, Supply Chain Analysts, Marketing Analysts, Financial Analysts, Operations Analysts, People Ops Analysts, and more.
Salesforce, Alteryx, Skyvia, Dataddo, Hevo, Xplenty, Parabola, Noteable, Looker, Astera, Funnel, Nifi, Microsoft Power BI, Qlik, Sisense, Datawrapper, Infogram
Automation & Application Platforms
Automation and application development platforms enable the creation of sophisticated workflows and custom software solutions without traditional coding. These versatile tools allow users to design and implement complex processes, create data pipelines, and develop tailored applications.
- Process Mapping & Visualization: users can transform abstract workflows into tangible, actionable diagrams, providing a visual overview of processes from start to finish. This aids in identifying inefficiencies and bottlenecks and serves as the blueprint for developing automated solutions to improve business efficiency.
- Task & Project Management: these platforms offer a comprehensive toolkit for planning, executing, and monitoring various business projects and tasks. With tools for assigning tasks, setting deadlines, tracking progress, scheduling, and resource management, users can better align project elements with business objectives.
- Workflow Automation: these tools provide a drag-and-drop or similarly user-friendly interface for designing workflows, integrating various business applications, and automating tasks like data entry, approvals, and email responses.
- Rule-based Logic and Conditional Workflows: users can set up rules and conditions that trigger specific actions within workflows, adding flexibility to the automation process. This capability is crucial for tailoring workflows to specific business rules, such as routing approvals based on project value, escalating issues based on severity, or triggering different actions based on customer responses.
- No-Code & Low-Code Development: these platforms empower users to rapidly develop and deploy applications and automations using low-code and no-code tools, which enable quick prototyping and testing, making development accessible for all business users.
- Templates: related to the ease of no-code and low-code development, some of these platforms offer templates for applications and automations for common business processes. They eliminate the need to start from scratch, making it easy for users to create applications and workflows tailored to their specific needs.
- Automating Patent Application Processes: a biotech company automates the workflow for preparing and submitting patent applications for new discoveries, ensuring timely filings.
- Automating Document Verification: an aerospace company implements a workflow to automatically verify the accuracy and compliance of technical documents against industry regulations.
- Automating Inventory Replenishment: a robotics manufacturer establishes an automated workflow for inventory management, triggering reorder processes when stock levels of critical components drop below a certain level.
- Optimizing Project Approval Processes: a nanotechnology research institute automates the workflow for project proposals and approvals, streamlining the review process.
- Streamlining Supplier Onboarding: a launch services startup automates the supplier onboarding process, efficiently managing contracts, quality checks, and integration into supply chains.
- Employee Training Portal: an AI research company builds a training portal application to onboard new employees with specialized courses and material on AI technologies and company protocols.
- Clinical Trial Management Application: a biopharmaceutical firm builds a custom application to manage clinical trials, tracking participant data, results, and ensuring compliance with regulatory standards.
- Contract and Legal Document Management: a biotech startup creates a system for managing legal documents, contracts, patent filings, and compliance paperwork.
- Inventory and Asset Management: a photonics company develops an internal application for inventory management, tracking assets, ordering supplies, and predicting future inventory needs.
- Customer Feedback Analysis Tool: an edge computing solutions provider creates an application to systematically collect and analyze customer feedback for product improvements and troubleshooting.
Product Managers, Project Managers, Business Analysts, Systems Analysts, QA Analysts, Data Architects, Application Developers, Account Managers, and others.
Workflow Automation:
SS&C Blue Prism Intelligent Automation Platform, Asana, Digibee, Anvil, Automate.io, BPLogix, Bryter, Clappia, Coda, Decisions, DronaHQ, Flowfinity, Formstack, Integromat, Kissflow, Kintone, Ninox, ClickUp, Miro, Knack, Pega, Nintex, Makerpad, Notion, Y42, Pipefy, Pneumatic, Praxie, Syncari, Workato, Zapier, Trackvia, Stackby, N8N.io, Tray.io, Monday
Enterprise Applications:
OutSystems, ServiceNow App Engine, Spring Boot, Airtable, HCL Domino, Microsoft Power Apps, Appian, Toca.io, Hatch Apps, Caspio, AppSheet, Bizago, Zudy, Mendix, Unqork, Jitterbit, Superblocks, Pega Platform
Getting Started with Platforms
By following these steps, you can strategically approach learning no-code platforms, focusing on those most relevant to your career goals. Remember, the key is to understand the underlying principles and functionalities rather than trying to master every single platform. Your mathematical background gives you a strong foundation for quickly grasping new tools and applying them effectively in various contexts.
Compile a comprehensive list of all the software and analytical tools you used during your academic work. You might be surprised by the length of this list — math majors often have more practical experience than they realize. Instead of focusing on the specifics of each tool, concentrate on their broader functionalities:
- What types of tool(s) did you use?
- What did they allow you to accomplish?
- What similarities exist between these tools and the platforms used in non-academic settings? Note that in some cases, tools used in academia are also used in industry.
By distilling your experience into core competencies and functionalities, you'll uncover valuable skills that translate directly to professional environments. Once you've identified these transferable skills, make sure to highlight them prominently on your resume. This approach allows you to showcase your practical abilities and demonstrate how your academic experience has prepared you for professional roles, even if you haven't used the exact platforms mentioned in job listings.
Start by clarifying the types of roles you want to pursue and the problems you'd like to solve. With this focus in mind, begin exploring job postings for these positions. As you review them, compile a list of platforms that are frequently mentioned. If this list becomes extensive, shift your attention to the types of platforms and their core functionalities, rather than focusing on specific brands.
While some employers may require experience with particular platforms, most are more interested in your ability to perform tasks related to the role's core functions. In these cases, experience with one platform often translates easily into competence with similar ones. This approach allows you to target your learning efforts more efficiently, focusing on building transferable skills rather than mastering every individual platform.
By understanding the broader categories and functionalities of no-code tools used in your desired roles, you'll be better prepared to adapt to various platforms and demonstrate your value to potential employers.
After assessing your experience and goals, it's time to determine how to gain or showcase the skills necessary for your desired role. Your approach will depend on your current level of experience.
For those with significant experience:
- Review projects and problems you've solved using these tools
- Highlight the types and functionalities of tools you've used on your resume
- Prepare to discuss these experiences in interviews, demonstrating how you can apply similar problem-solving skills with related tools in professional settings
For those new to no-code & low-code platforms, or with limited experience:
- Focus on what you minimally need to accomplish to demonstrate competence
- Create a personal syllabus with concrete, achievable goals
- Seek out open-source resources or free tutorials for tools similar to those used in your target roles, or
- If you've identified a specific platform crucial for your desired role, explore paid training options, or
- Look into free courses with paid certificates offered by platform providers
Remember, the goal is to build a foundation of skills that will allow you to adapt to various tools and solve real-world problems. Whether you're showcasing existing skills or developing new ones, focus on how your mathematical background enhances your ability to leverage these platforms effectively.
Find Code-Free Careers
While mathematicians are often familiar with technical and applied positions in industry, many are less aware of the wealth of strategic, analytical, and operational roles for which their skills are highly valuable. To broaden the horizon of opportunities, we've curated a list of over 20 career tracks that require minimal to no coding. These roles are grouped into five categories based on their primary functions.
Each of these career tracks offers unique opportunities to leverage your mathematical expertise without extensive coding requirements. To explore these roles in greater depth and find the paths that align with your interests and strengths, visit our Career Tracks page [LINK]. There, you'll find detailed information about each role, including typical responsibilities, required skills, and potential career trajectories.
After identifying a career track that aligns with your interests and skills, it's crucial to carefully analyze job postings to determine the level of technical knowledge required. When searching for code-free or low-code positions, pay attention to these key indicators:
- Platform-specific language: Jobs requiring minimal coding often mention specific analytical platforms or tools by name (e.g., Tableau, Power BI, Alteryx).
- Emphasis on analytical skills: Look for phrases like "data analysis," "statistical modeling," or "business intelligence" without accompanying coding requirements.
- Focus on problem-solving: Postings that highlight critical thinking and problem-solving skills over technical prowess may be more suitable for code-free roles.
- Absence of programming languages: If a job doesn't list specific programming languages (like Python, R, or SQL) as requirements, it's more likely to be a low-code or no-code position.
Conversely, more technical jobs typically emphasize programming languages, software development methodologies, and specific coding skills. By honing your ability to discern these differences in job descriptions, you can more effectively target positions that match your desired level of technical involvement.
Keep in mind, some postings may list coding skills as "preferred" rather than "required." In these cases, your strong mathematical background and analytical skills may still make you a competitive candidate, especially if you demonstrate willingness to learn.
When crafting your resume and preparing for interviews, emphasize skills that are readily transferable across different platforms and industries. Your ability to quickly learn and adapt to new tools is often more valuable than deep expertise in a single platform. Highlight examples that demonstrate your adaptability and rapid learning capacity. While some roles may require specific platform expertise, many employers prioritize candidates who can swiftly grasp new technologies and apply their problem-solving skills across various tools.
Showcase how your mathematical background equips you with a strong analytical foundation, enabling you to efficiently master new platforms and apply them to solve complex problems. This approach positions you as a versatile candidate capable of evolving with the changing technological landscape.
Don't let a lack of experience with specific tools deter you from applying to an interesting job. Instead, approach knowledge gaps as opportunities to demonstrate your adaptability and learning capacity.
By addressing knowledge gaps proactively, you demonstrate valuable traits like self-awareness, initiative, and adaptability. These qualities, combined with your strong mathematical foundation, can make you an attractive candidate even when you don't tick every box in the job description.
Future-Proof Your Career
In an era of rapid technological advancement, future-proofing your career is less about predicting specific changes and more about cultivating adaptability and a problem-solving mindset.
By focusing on these principles, you can build a career that not only withstands technological changes but thrives on them. Remember, the goal is not to predict the future, but to create a flexible, resilient career foundation that allows you to adapt and grow with whatever changes come your way.
Here are some ways to position yourself for long-term success:
The key to long-term career adaptability lies in orienting yourself towards the problems you're passionate about solving, rather than becoming overly attached to specific tools or technologies. While we can't predict the exact nature of future innovations, we can be certain that new technologies will continually emerge. By adopting a problem-driven approach to your career, you position yourself to remain relevant and valuable regardless of technological shifts.
This mindset allows you to: adapt quickly to new tools that address familiar problems; transfer your skills across different domains and industries; identify innovative solutions by applying mathematical thinking to diverse challenges; and stay motivated by focusing on the impact of your work, not just the methods.
Remember, tools are means to an end. By keeping your focus on the problems you want to solve, you'll naturally gravitate towards learning and mastering the most effective tools for the job, whatever they may be in the future. This problem-centric approach ensures that your skills and knowledge evolve in tandem with the changing technological landscape, maintaining your relevance and effectiveness throughout your career.
Developing a mindset that views technological change as an opportunity rather than a threat is crucial for long-term career success. By reframing change as a chance to tackle bigger problems or approach familiar challenges in innovative ways, you'll be better positioned to identify and create impactful solutions.
By embracing continuous learning and adaptability, you'll not only remain at the forefront of possible solutions but also develop the resilience to thrive in an ever-changing technological landscape. This approach ensures that you're always ready to leverage new opportunities and tackle emerging challenges, keeping your skills relevant and your career trajectory on an upward path.
Self-awareness of your fundamental skills and strengths forms the bedrock of a career that is both fulfilling and resilient. By thoroughly understanding your core competencies, you position yourself to be not only more competitive in the job market but also more adaptable and satisfied in your professional life.
Your core mathematical competencies are transferable across many fields and technologies. By maintaining a clear understanding of these fundamental skills, you'll be better equipped to spot diverse opportunities, adapt to technological changes, and apply your expertise in innovative ways throughout your career.
Your unique perspective, honed through mathematical training, allows you to see patterns and possibilities that others might overlook. By continuously exploring new applications for your skills, you can create opportunities that are resistant to automation and uniquely valuable in the marketplace.
Mathematicians are among the most creative and innovative thinkers in any field. This innate creativity becomes a powerful asset in a rapidly evolving technological landscape. Even if certain aspects of mathematical work become automated, your ability to apply mathematical thinking in novel ways remains invaluable.
At its core, mathematical thinking is a profoundly human endeavor. It's our unique way of questioning, understanding, and describing the structure of the universe and our experience within it. This fundamental aspect of mathematics transcends specific tools or technologies and is likely to remain invaluable regardless of future advancements.
The practice of organizing information and finding patterns across scales, from the nanoscopic to the galactic, is a uniquely human capability. While machines excel at processing data and executing algorithms, the human mind's ability to contextualize, interpret, and derive meaning from mathematical concepts remains unparalleled.
As you navigate your career, remember that your mathematical training equips you with more than just technical skills. It provides a powerful lens through which to view and understand the world. This perspective, combined with creativity and a deep passion to build enduring solutions ensures that the human element in mathematics will continue to be essential, driving innovation in ways that complement and guide technological advancements.
Recap
As we conclude this guide, let's recap the key insights that will empower you to build a resilient and fulfilling career leveraging your mathematical expertise:
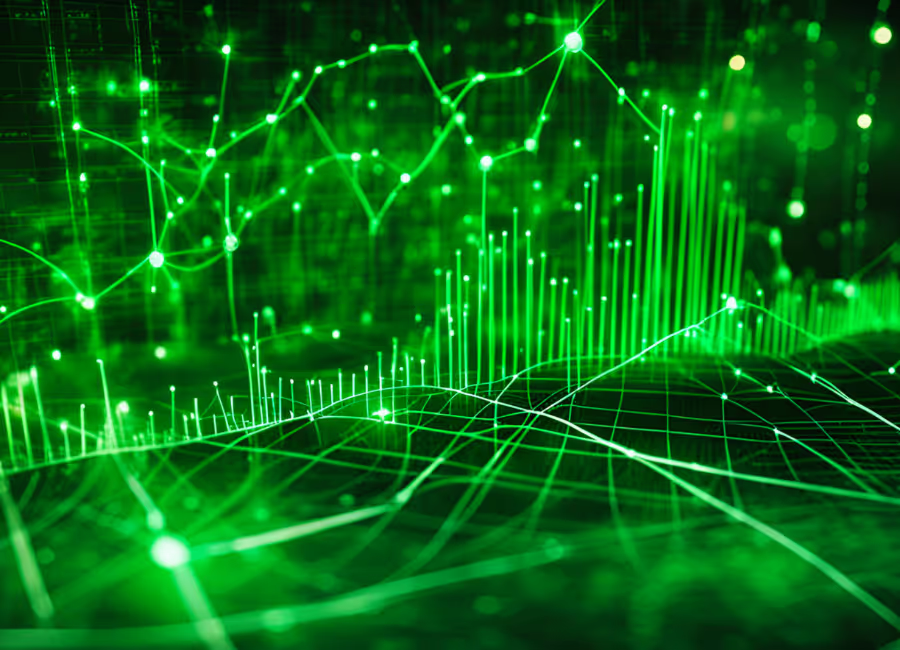
Leverage Your Versatility
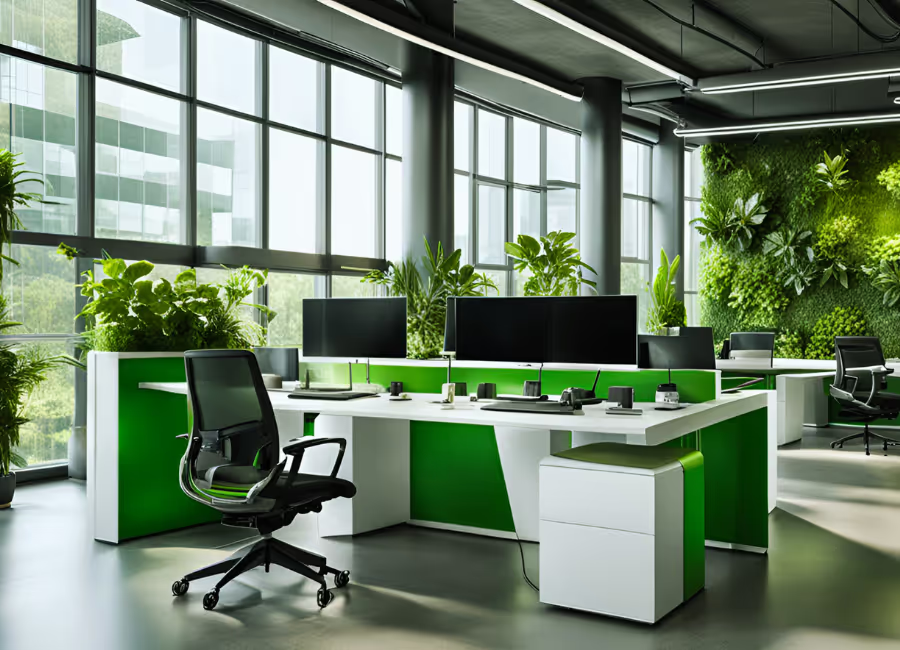
Consider Nontechnical Opportunities

Adopt a Problem-Driven Approach

Know Yourself and Your Aspirations
Additional Resources
Start your deep tech journey with our resource collection